Synchronization in Dynamical Systems and Complex Networks and its Application
Prof. Jürgen Kurths // Potsdam Institute for Climate Impact Research and Humboldt University Berlin, Department of Physics
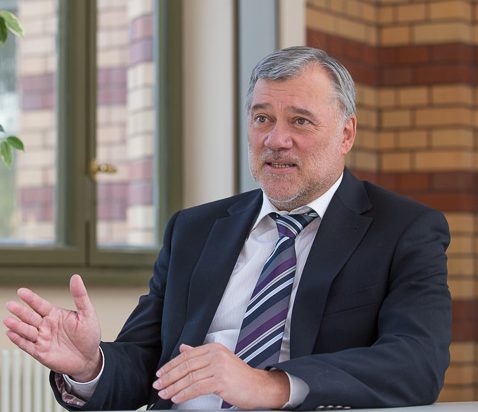
Abstract:
Synchronization phenomena are abundant in nature, science, engineering and social life, such as in organ pipes, fireflies and even in the mechanics of bridges. But synchronization was first recognized by Christiaan Huygens in 1665 for coupled pendulum clocks; this was the beginning of nonlinear sciences. In the last two decades, this concept has been successfully extended to more complex systems, as coupled chaotic oscillators and even distributed systems as complex networks. Complex networks were firstly studied by Leonhard Euler in 1736 when he solved the Königsberger Brückenproblem. Recent research has revealed a rich and complicated network topology in various model systems as well as in several fields of applications, such as transportation and social networks, or the WWW.
In this talk synchronization phenomena on active complex networks will be discussed. I report the advances in the comprehension of synchronization phenomena when oscillating elements are constrained to interact in a complex network topology. I also present new emergent features coming out from the interplay between the structure and the function of the underlying patterns of connections. The classic local stability in dynamic networks is compared to the new basin stability concept. Finally, several applications of synchronization in complex networks from human brain via power grids to climate are given.
Coupling and Synchronization Analysis in the Earth’s Climate System: From continuous dynamics to extreme events and back
Dr. Reik V. Donner // Magdeburg-Stendal University of Applied Sciences / Potsdam Institute for Climate Impact Research
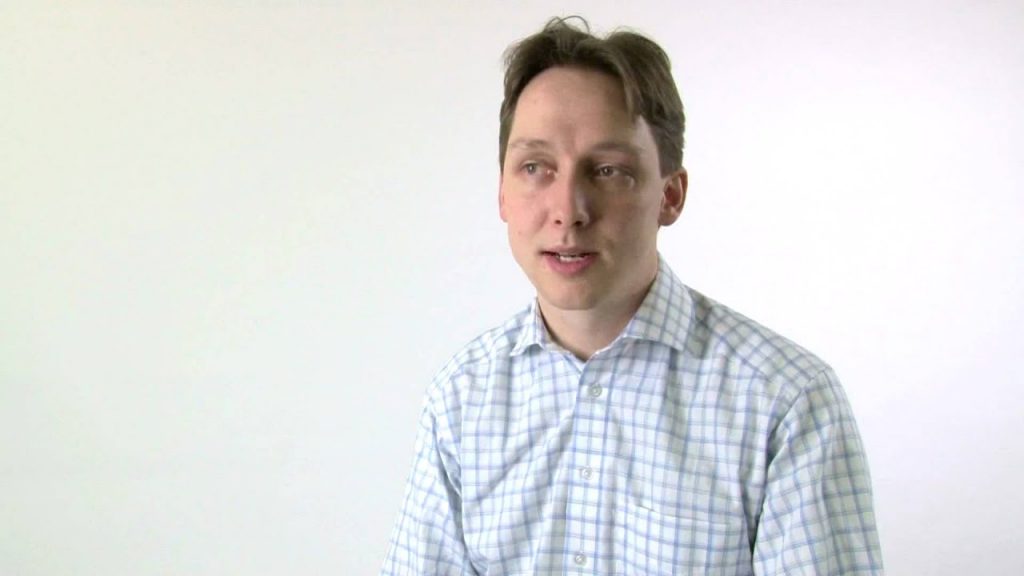
Abstract:
For better understanding how different variables and patterns interact which each other to govern regional and global climate dynamics, advanced approaches from complex system science have been recently demonstrated to provide versatile and widely applicable tools complementing information provided by classical methods of statistical climatology. In this lecture, I will elaborate on three selected approaches developed and successfully applied in our recent work.
The first part will summarize recent achievements around the application of synchronization analysis and complex network methods to understanding different types of large-scale climate phenomena based on the thorough analysis of continuous time series of climate variability. As a particular application, I will discuss the differential fingerprints of different types of El Nino—Southern Oscillation (ENSO) phases in global surface air temperature fields and how they can be used for obtaining a meaningful classification of ENSO flavors in terms of their global imprints.
The second main topic of this lecture will be devoted to the inference of statistical relationships between event sequences, such as the timings of extreme precipitation events, which may reflect their dynamical coupling. I will introduce the concept of event synchronization along with some of its successful applications in regional climate as well as neuroscience studies, and contrast it with the so-called event coincidence analysis, which provides additional options for studying synchronicity of events in more depth. Differences between both approaches are highlighted based on case studies for the South American and Asian monsoon systems.
Finally, I will elaborate on the problem of causal inference based on multivariate climate data, introducing the concept of causal effect networks allowing for disentangling the complex interactions between different climate patterns. The power of this technique will be illustrated by some recent successful applications aiming at better understanding the interplay between the Indian summer monsoon and its tropical as well as extratropical drivers at different intraseasonal time scales.